Downloads
Important Notice, the authorized accounts that do not provide true and valid basic institute and group information will be deactivated after one month.
Executable file – CATKINAS
ReadMe – CATKINAS
Examples – CATKINAS
Donate to CATKINAS
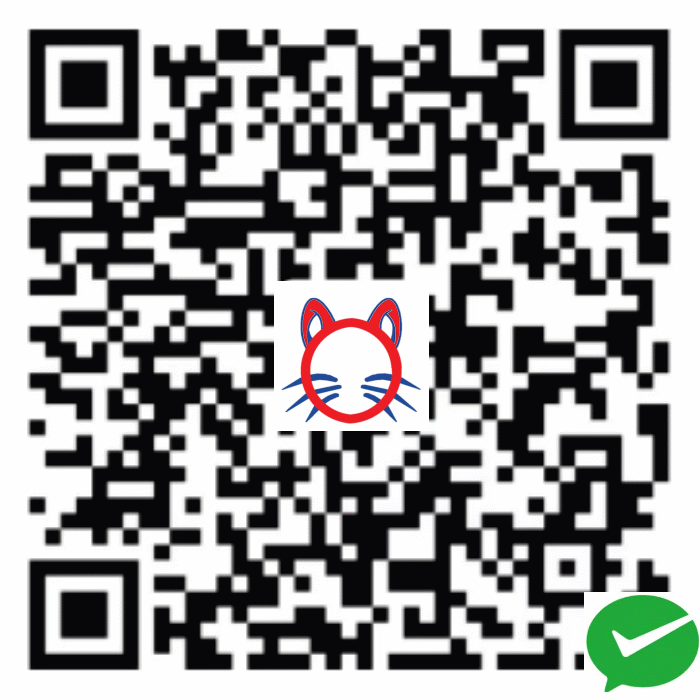
This software is for academic and noncommercial purpose. You need to login/register before you can download or view attachments, or contact us via jfchen@ecust.edu.cn or hfwang@ecust.edu.cn, with your basic institute and group information provided.
Other developed software
CATIDPy: Catalyst Inverse Design for Python
We develop an automated and oriented workflow that utilizes genetic-algorithm-based global optimization method to guide the on-the-fly Density FunctionalTheory (DFT) calculations without user intervention and experts’ domain knowledge. This general workflow can be expandable and has significant application prospects for designing catalyst with one or more criteria. These criteria can be thermodynamic descriptors, kinetic descriptors and even some physical indicators.
Machine Learning Potential
Machine learning potential for Ru-O
The global dataset was constructed iteratively via the self-learning protocol run with machine learning potential (MLP) and monitored by the density functional theory (DFT) calculations, covering more than 146 thousand surface structures on the target potential energy surface (PES). Specifically, they include different Ru/O compositions and layers mainly evolved from RuO2(110) and different atom-number unit cells (~40-145) to ensure the structural diversity. The mean absolute error (MAE) and root-mean-square error (RMSE) for the entire training data with respect to energy are 4.1 and 5.8 meV atom-1, respectively. In terms of force prediction accuracy, the values are 0.15 eV Å-1 for MAE and 0.21 eV Å-1 for RMSE.